Automated Machine Learning (AutoML) systems are an important part of the machine learning engineering “toolbox”. However, existing solutions often require substantial amounts of compute, and therefore extended running times, to achieve competitive performance.
In this post, we evaluate Plexe, an AutoML framework designed to deliver competitive or superior predictive performance while demanding fewer computational resources compared to other popular frameworks. We use as our baseline performance results published in the 2024 paper by Gijsbers et al. In this post, we frequently refer to their methodology and findings, so we encourage you to check out their work.
Across a set of 20 benchmark datasets, relative to the AutoML tools we are evaluating against, Plexe consistently achieves superior or competitive prediction accuracy, while converging to a solution in a shorter wall time on comparable hardware.
While we are not yet ready to release Plexe itself to the world, the benchmark experiments themselves are fully reproducible, with code and data publicly accessible at GitHub - plexe-ai/plexe-results. We plan to release Plexe in the coming months.
Experimental Design
Baseline Selection
We compare against leading AutoML frameworks:
AutoGluon(B)
H2O AutoML
LightAutoML
FLAML
AUTO-SKLEARN
MLJAR
TPOT
Evaluation Protocol
We evaluate Plexe following the same protocol described in the paper by Gijsbers et al:
We selected 20 datasets from the OpenML benchmark suite
We apply a 1-hour maximum runtime cutoff per dataset
Standard metrics (AUC for classification, RMSE for regression)
Hardware Configuration
All experiments were conducted on standardized cloud infrastructure:
8 vCPUs
30GB RAM
Standard cloud instance (Digital Ocean)
This is comparable to the hardware setup used by Gijsbers et al in their paper.
Results
Benchmark Performance
Results across the OpenML benchmark suite show consistent performance improvements:
Dataset | Size | Features | Domain | Plexe | AutoGluon(B) | H2O AutoML | LightAutoML | FLAML | AUTO-SKLEARN | MLJAR(B) | TPOT |
kr-vs-kp | 3,196 | 36 | Games (Chess) | 1.000 | 1.000 | 1.000 | 1.000 | 0.961 | 1.000 | 0.999 | |
adult | 48,842 | 14 | Census | 0.933 | 0.932 | 0.931 | 0.932 | 0.932 | 0.930 | 0.927 | |
bank-marketing | 45,211 | 16 | Finance | 0.938 | 0.941 | 0.938 | 0.940 | 0.937 | 0.939 | 0.935 | |
phoneme | 5,404 | 5 | Audio | 0.971 | 0.968 | 0.968 | 0.966 | 0.972 | 0.963 | 0.969 | |
MiniBooNE | 130,064 | 50 | Physics | 0.987 | 0.989 | 0.987 | 0.988 | 0.987 | 0.987 | 0.987 | 0.982 |
Australian | 690 | 14 | Finance | 0.951 | 0.941 | 0.935 | 0.946 | 0.938 | 0.931 | 0.943 | 0.939 |
kc1 | 2,109 | 21 | Software | 0.838 | 0.840 | 0.829 | 0.831 | 0.841 | 0.843 | 0.828 | 0.844 |
blood-transfusion | 748 | 4 | Medical | 0.768 | 0.758 | 0.764 | 0.753 | 0.730 | 0.747 | 0.724 | |
qsar-biodeg | 1,055 | 41 | Chemistry | 0.952 | 0.942 | 0.939 | 0.934 | 0.930 | 0.931 | 0.932 | 0.926 |
Click prediction | 39,948 | 11 | Marketing | 0.721 | 0.710 | 0.701 | 0.728 | 0.723 | 0.697 | 0.709 | 0.715 |
Steel plates* | 1,941 | 27 | Industrial | 0.464 | 0.464 | 0.490 | 0.478 | 0.482 | 0.516 | 0.468 | 0.509 |
Airlines | 539,383 | 7 | Travel | 0.725 | 0.732 | 0.732 | 0.727 | 0.731 | 0.726 | 0.731 | 0.722 |
Numerai | 96,320 | 21 | Finance | 0.532 | 0.531 | 0.531 | 0.531 | 0.528 | 0.530 | 0.530 | 0.527 |
Gina | 3,153 | 970 | Computer Vision | 0.992 | 0.991 | 0.991 | 0.990 | 0.992 | 0.990 | 0.991 | 0.988 |
credit-g | 1,000 | 20 | Finance | 0.798 | 0.796 | 0.779 | 0.796 | 0.788 | 0.778 | 0.791 | |
Bioresponse | 3,751 | 1,776 | Biology | 0.882 | 0.886 | 0.887 | 0.884 | 0.884 | 0.873 | 0.882 | 0.880 |
Vehicle | 846 | 18 | Physical | 0.955 | 0.951 | 0.942 | 0.947 | 0.944 | |||
Sylvine | 5,124 | 20 | Physical | 0.994 | 0.990 | 0.990 | 0.988 | 0.991 | 0.991 | 0.992 | 0.992 |
Wilt | 4,839 | 5 | Nature | 0.992 | 0.995 | 0.992 | 0.994 | 0.991 | 0.994 | 0.999 | 0.996 |
pc4 | 1,458 | 37 | Software | 0.956 | 0.952 | 0.948 | 0.950 | 0.950 | 0.942 | 0.951 | 0.944 |
Note: *Steel plates uses logloss metric (lower is better)'-' indicates framework failed to complete or produce valid results
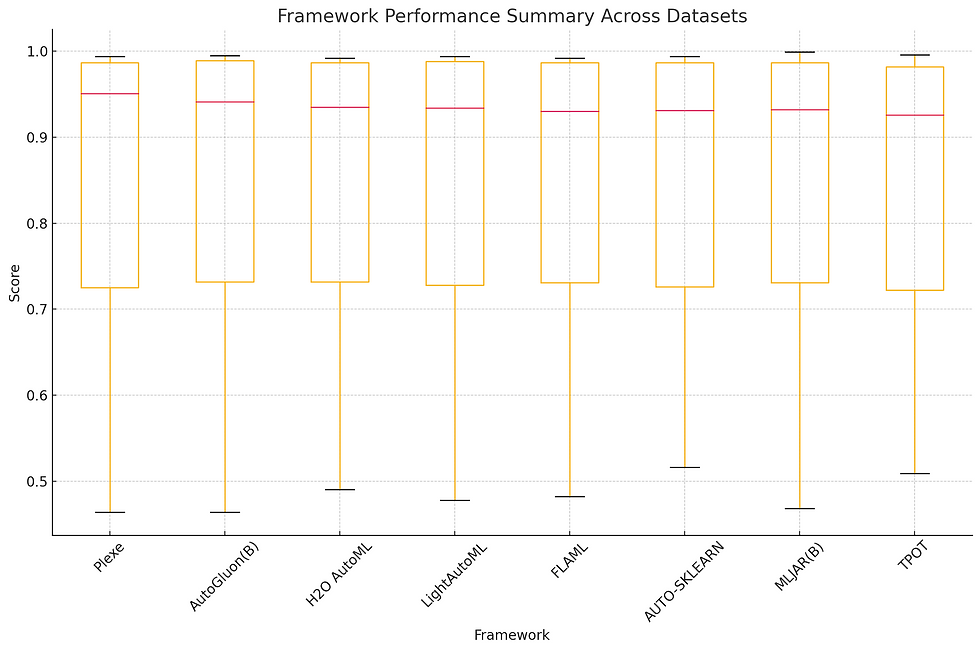
Computational Efficiency
In the AutoML benchmark experiment, while a one-hour budget was set for each framework's training, not all frameworks consistently used the full time. In fact, there were instances where frameworks finished well before the allotted hour, indicating variability in processing time requirements across frameworks and datasets. Additionally, certain frameworks occasionally exceeded the time budget by a few minutes, though significant overruns were rare and generally controlled within an acceptable range of leniency. In Plexe’s case, all best solutions were found well within the 1 hour limit, with 75% finishing in less than 30 minutes.
External Validation: Kaggle Competitions
Performance on Kaggle competitions provides independent validation and an example for a situation where Plexe can be immediately useful:
Domain | Competition | Plexe’s Percentile Rank | Plexe’s absolute Rank |
Finance | 73.14% | 765/2845* | |
Real Estate | 83.12% | 800/4735* | |
Analytics | 85.73% | 2200/15422* | |
Retail | 32.8% | 447/665 | |
Analytics | 61.5% | 595/1549* | |
Social Media | 62.5% | 377/1008* | |
Cybersecurity | 61.8% | 2113/5534* | |
Healthcare | 83.6% | 47/287* | |
Retail | 33.7% | 11183/16863 | |
Healthcare | 63.7% | N/A - closed competition | |
Urban | 83% | N/A - closed competition |
Note: * represents competition ongoing at the time of submission
Side note: Test set accuracy for the Titanic Survival dataset reported by MLJar (running 4 hours job on m5.24xlarge machine (96CPU, 384GB RAM)): 77.99% whereas Plexe reported an accuracy of 84.51%.(with a 1 hour limit running on the same hardware as defined on the top of this blog post)
Discussion
While these early results are only indicative, we do find them encouraging. In particular, we observed:
Superior performance relative to established tools on 12/20 benchmark datasets
Competitive performance (within 0.005) on remaining datasets
Consistent results across diverse problem types
We acknowledge that the experiment has a number of limitations, which constrains the conclusions we can draw:
Limited to tabular data
Limited to 20 (OpenML) + 11 (Kaggle) tasks, all of which have “small” datasets
Evaluation restricted to 1-hour time budgets
Benchmark suite could be expanded to include more recent datasets
Nonetheless, we feel these initial results warrant further work on the tool. There are several directions that we want to explore:
Extension to unstructured data types
Support for deep learning models
Expansion of benchmark suite with additional datasets
Conclusion
Our empirical evaluation of Plexe’s early prototype suggests that the tool is capable of achieving competitive or superior performance compared to current AutoML frameworks, while reducing computational requirements. This provides an encouraging foundation for us to continue building upon, and we plan to release a fully fledged beta to the public in the coming months. Stay tuned for updates!
References
Gijsbers, P., Bueno, J., Coors, S., LeDell, E., Poirier, S., Thomas, J., Bischl, B., & Vanschoren, J. (2023). AMLB: An AutoML Benchmark. 22-0493.pdf, benchmarking results and framework evaluation.
Comments